Thomas J. Vandal, Ph.D.
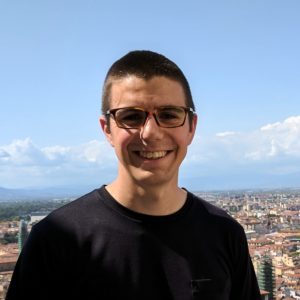
Check out my company Zeus AI where we use machine learning to generate continuous and global weather observations and foreasts in real-time.
My research is at the intersection of machine learning, computer vision, Earth science, and high-performance computing. I am interested in machine learning applications to our ever growing datastores of spatio-temporal Earth science observations, forecasts, and projections for climate mitigation and adaptation. Recent projects have included developments of novel deep learning methods for atmospheric wind, climate downscaling, emulation, virtual sensing, and cloud tracking.
Bio
Thomas is a co-founder and the chief executive officer of Zeus AI and a scientist at NASA Ames/BAER Institute. He is a Principle Investigator (PI) in the NASA ROSES geostationary satellite program studying machine learning for tracking atmospheric motion in satellite imagery. Thomas earned a Ph.D. in Interdisciplinary Engineering from Northeastern University in 2018 while working in the Sustainability and Data Sciences Lab advised by Auroop R. Ganguly. During graduate school his research won runner-up best paper award at SIGKDD 2017, outstanding graduate researcher at Northeastern University in 2018, and served as an elected student member of the committee on Artificial Intelligence Applications to Environmental Science for the American Meterological Society (AMS). Prior to graduate school he worked at startups in the Boston area, including the MIT Media Lab spin-out Affectiva. He completed his bachelors in mathematics at the University of Maryland College Park in 2012.
Projects
NASA Small Business Innovation Research (SBIR) Phase I (2022-2023)
Title: Predicting 3D atmospheric structure from geostationary satellites
PI: Vandal
Company: Zeus AI, Inc.
NASA ROSES Earth Science Research from Geostationary Satellite Program (2020-2023)
Title: Quantifying Earth Process Dynamics with Optical Flow on Geostationary Satellite Imagery
PI: Vandal
Institutes: Ames, Goddard Space Flight Center (GMAO)
NASA ROSES Applied Sciences Water Resources Program (2022-2025)
Title: Remote-sensing data driven Artificial Intelligence for precipitation-Nowcasting (RAIN)
PI: Auroop Ganguly, Co-I’s: Vandal and Duffy
Institutes: Northeastern, Ames, DOE ORNL, Tennessee Valley Authority
Recent Work
(August 2022) Publication Multi-sensor machine learning to retrieve high spatiotemporal resolution land surface temperature in IEEE Access.
(August 2022) Presented our paper Dense Feature Tracking with Deep Optical Flow at SIGKDD 2022 in Washington, DC.
(May 2022) NASA SBIR Phase I is selected for Zeus AI.
(April 2022) Paper titled “A framework for deep learning emulation of numerical models with a case study in satellite remote sensing” published in IEEE TNNLS Special Issue on Deep Learning in Earth and Planetary Geosciences.
(January 2022) Organized a session on “Deep Learning for LEO-GEO Satellite Observations” at the 21st Conference on Artificial Intelligence for Environmental Science.
(December 2021) Invited talk presented at AGU’s fall meeting and the Indian Symposium on Machine Learning (IndoML)
(December 2021) Presented at the Indian Symposium on Machine Learning (IndoML).
(December 2021) Presented at the AGU Fall Meeting on Physics Guided Optical Flow.
(September 2021) Presented at the The 3rd NOAA Workshop on Leveraging AI in Environmental Sciences, slides.
(August 2021) Paper titled “Temporal Interpolation of Geostationary Satellite Imagery With Optical Flow” published in IEEE TNNLS Special Issue on Deep Learning in Earth and Planetary Geosciences.
(June 2021) Paper titled “Spectral Synthesis for Geostationary Satellite-to-Satellite Translation” published in IEEE TGRS Paper.
(August 2020) Awarded best spotlight presentation at 1st ACM SIGKDD Workshop on Deep Learning for Spatiotemporal Data, Applications, and Systems.
(May 2020) Paper accepted to KDD 2020 research track titled “High-Dimensional Similarity Search with Quantum-Assisted Variational Autoencoder” Paper
(May 2020) NASA ROSES proposal selected by to develop optical flow methods for tracking atmospheric motion. Selections.
Selected Publications
Vandal, T., Duffy, K., McCarty, W., Sewnath, A., & Nemani, R. (2022). “Dense feature tracking of atmospheric winds with deep optical flow”, Proceedings of the 28th ACM SIGKDD Conference on Knowledge Discovery and Data Mining (Research track, Oral, ~8% acceptance). [Paper], [Code].
Duffy. K., Vandal, T., & Nemani, R. (2022). Multi-sensor machine learning to retrieve high spatiotemporal resolution land surface temperature. IEEE Access. [Paper] [Code]
Duffy. K., Vandal, T., Wang, W., Nemani, R., & Ganguly, A. R. (2022). A framework for deep learning emulation of numerical models with a case study in satellite remote sensing. IEEE Transactions on Neural Networks and Learning Systems (TNNLS). [Paper] [Code]
Vandal, T. & Nemani, R. (2021). “Optical Flow for Intermediate Frame Interpolation of Multispectral Geostationary Satellite Data”. IEE Transactions on Neural Network and Learning Systems (TNNLS). Special issue on Special Issue on Deep Learning for Earth and Planetary Geosciences. [Paper] [Code] [Video].
Vandal, T., McDuff, D., Wang, W., Duffy, K., Michaelis, A., & Nemani, R. (2021) “Spectral Synthesis for Geostationary Satellite-to-Satellite Translation”. IEEE Transactions on Geoscience and Remote Sensing. [Paper] [Code]
Vandal, T. & Nemani, R. (2020). “Optical Flow for Intermediate Frame Interpolation of Multispectral Geostationary Satellite Data”. In Proceedings of 1st ACM SIGKDD Workshop on Deep Learning for Spatiotemporal Data, Applications, and Systems (DeepSpatial ’20). [Paper]
Gao, N., Wilson, M., Vandal, T., Vinci, W., Nemani, R., & Rieffel, E (2020). “High-Dimensional Similarity Search with Quantum-Assisted Variational Autoencoder,” Proceedings of the 26th ACM SIGKDD Conference on Knowledge Discovery and Data Mining (Research Track, 16% acceptance rate). [Paper]
Vandal, T., Kodra, E., Ganguly, S., Dy, J., Nemani, R., & Ganguly, A (2018): “Quantifying Uncertainty in Discrete-Continuous and Skewed Data with Bayesian Deep Learning,” Proceedings of the 24rd ACM SIGKDD Conference on Knowledge Discovery and Data Mining, 1663-1672. (Research Track, 18% acceptance rate) [Paper], [Code].
Vandal, T., Kodra, E., Ganguly, S., Michaelis, A., Nemani, R., & Ganguly, A. (2017). “DeepSD: Generating high resolution climate change projections through single image super-Resolution,” KDD 2017, Proceedings of the 23rd ACM SIGKDD Conference on Knowledge Discovery and Data Mining, 1663-1672. (Runner-Up BEST PAPER Award in Applied Data Science Track, 9% oral acceptance rate). [Paper] [Code].